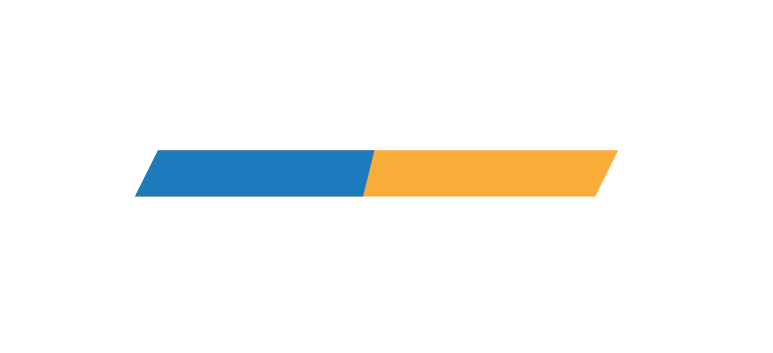
Artificial Intelligence in Banking
The Meaning of Artificial Intelligence
In concept, Artificial Intelligence (AI) has been around for decades, ever since John McCarthy defined it as “the science and engineering of making intelligent machines”. But it is only lately that AI technology has undergone rapid evolution and consequently sparked significant interest among enterprises in virtually every industry.
Artificial Intelligence in Banking
AI driven startup ventures are looking to redefine banking, and progressive banks have launched AI based pilots, be it in the space of customer services, fraud management, or credit scoring, among others. These ventures and pilots have gained prominence because AI powered banking is viable now and it is also seeing acceptance among end consumers. All this has led to banking business and technology leaders agreeing that artificial intelligence is among the hottest banking trends that will reshape banking in 2020.
What are the 9 major financial institutions that will be impacted by AI?
Central Banks , Retail and Commercial Banks, Internet Banks, Credit Unions, Savings and Loan Associations, Investment Banks and Companies, Brokerage Firms, Insurance Companies, Mortgage Companies.
Artificial intelligence in financial services
How is AI being used in finance?
Banks can use AI technology to stay in compliance and identify fraud. Artificial intelligence helps financial services companies make money by enhancing the accuracy of trading and by making wealth management more efficient.
Although the banking and financial services sector is showing interest in AI, research found that it is clearly not very mature in its journey to adoption. This is surprising considering that a financial service is a data intensive business.
The survey titled – Artificial Intelligence in Financial Services by FINEXTRA and QUANTEXA, which covered respondents from 10 vertical groups, tried to assess their respective progress in the AI journey with the help of a maturity index. On an average, most banks are explorers, with AI related skills on the increase and more initiatives planned in the coming 12 months.
Current and Future Use Cases
Confidence across the industry is high that AI will play an important role in the short and medium term in areas as diverse as operational efficiency, risk management, fraud prevention and customer insights. According to the survey, only 10% of respondents said there are no significant plans to use AI in their department or organization. 54% are already pursuing or planning major adoption or kicking off a programmer, while 30% are still in an experimental phase. As more and more data is being processed in real time from within and outside of financial organizations it is no surprise that they are looking to leverage this to improve their operational efficiency.
In fact, operational optimization and compliance, surveillance and anti-money laundering were the most common use cases identified in this survey. Compliance and financial crime have proven to be functions with a particularly compelling business case for the adoption of AI techniques. This is largely due to the large staff requirements and high false positive alert rates for non-AI driven approaches, as well as increases in regulatory oversight and penalties.
However, these successes have only really come as a result of the aggregation of multiple data sources and providing context around customer behavior before applying analytical models to reduce the human effort in compliance review tasks. Instead of many staff focused on low level monitoring and appraisal they can instead turn to robotics and artificial intelligence, while focusing on up-skilling employees for higher value tasks. 17% of respondents indicated these optimization and compliance use cases are leading the pack, when they consider AI implementation in their business.
Other prominent use cases revolves around the customer; learning more about their behaviors, anticipating their needs and identifying opportunities, while making the on- boarding process more streamlined for new products and relationships, and improving service. Sales and marketing functions tend to be next on the agenda for those organizations that have already achieved some level of maturity in AI deployment for financial crime.
To be successful, use cases in customer insight and referral-based sales, need to be built on the same key ingredients that market leaders have used to drive success in their financial crime project, full context of data from multiple sources, and a network view of entities and transactions. With this in place, AI models can then be deployed to assist or suggest to a customer what product may make sense for them or to help a relationship manager work out if a prospect is good, receptive and how to find a route to effectively contact them.
Deployment Approaches, Cost and ROI
Moving AI beyond experimentation and into enterprise usage and acceptance can require significant investment. In some cases it can be easy to measure the return on this investment, post-implementation. Operational efficiency use cases, where outcomes have improved or stayed the same with reduced headcount, are obvious examples. But in other use cases – particularly new product delivery, improved risk management or customer service improvement, ROI can be harder to calculate. This survey found that 26% of respondents who had live deployments of any AI driven project had seen strong ROI or outstanding results with payback within months.
54% of the respondents have begun to see some return on their investment, with an anticipated multi-year payback time frame. But 20% have gone live with AI projects that have yet to deliver any return on the investment required to bring them to production. Interestingly, when asked about how they have predominantly managed their AI initiatives to date, 33% said they had built and deployed themselves through the internal teams. But this group made up more than half of those with projects that failed to deliver any ROI. The next most common deployment approach has been to create a platform for multiple use cases (20% of respondents) which shows desire to avoid the fragmentation of AI solutions and approaches that can occur when project initiation and approvals arrive from multiple sources within a large financial organization.
Much smaller numbers are going live purely with end-to-end packaged solutions (10%) or cloud providers (8%). Looking ahead in the next 12 months to mid-2020, survey found departmental budgets for AI projects were healthy across a range of sectors and organization sizes in the financial services industry. Twenty-nine per cent of respondents had departmental allocation of budget for these projects in excess of $1m.
A Closer Look at the AI Stack
- AI Foundation (Data and Analytics)
- AI Building Blocks
- Applied AI Solutions
AI Building Blocks
- Machine Learning
- Deep Learning
- Natural Language Processing (NLP)
- Speech Recognition
- Natural Language Generation (NLG)
- Visual Recognition
Machine Learning
Machine Learning is simply, the ability of computers and other smart machines to learn without being “taught” or programmed. It is at its heart, computer programs which change with data. The machine learning process has some similarities to data mining. Both search and identify patterns from data, but where data mining presents the findings to human beings for their attention, machine learning adjusts its program and actions on its own.
Popular applications of machine learning in banking
In banking, machine learning finds application in pretty much everything such as customer service, personal financial/ wealth management, and fraud/risk management.
Key Areas of AI Application
- Front Office
- Mid Office
- Back Office
Mid Office: Fraud Management
The figure gives a snapshot of how AI can be leveraged to redefine the process of fraud management spanning Anti Money Laundering (AML), Know Your Client (KYC), fraud detection and regulatory compliance.
Readiness of AI for Banking
Even though there is lot of excitement when it comes to implementation of AI for banking, there is still a lot of ground to cover when it comes the readiness of AI technologies and solutions for business.
As per analysis, following is the relative readiness of various technologies and applied AI solutions for the banking industry.
The excitement surrounding AI needs to be tinged with an air of caution as the technologies are supporting AI solutions is probably not as ready for real-time use as the hype suggests. It’s critical to set the realistic expectations for AI programs within the organization. While the AI technologies hold great promise, many of them are in the formative stages.
Conclusion
While the concept of Artificial Intelligence has been around for decades, it is only recently that the AI fantasy has started to turn into reality. Many of the technology pieces are already in place, albeit in varying stages of maturity. What’s left to do is stitch together these components to re-think banking processes and experiences. Many banks have made a start by incorporating several AI components into their processes and have experienced early results.
While the technology’s evolution is both rapid and impressive, banks, and indeed, all enterprises, should ground their adoption strategies and expectations in reality. Nevertheless, regardless of initial hiccups and gestation times for expected results, banks should waste no time in executing their AI plans.
Because AI is evolving so quickly, it does not allow banks the luxury of waiting till it matures, and those who do, risk never being able to catch up with the leaders. Quick movers have another advantage in that their AI systems will start learning earlier than others, and will therefore evolve faster as well.
With AI, the industry will go through a long voyage of re-imagining banking, spanning several years, many milestones and at least a few challenges.
References
https://www.edgeverve.com/artificial-intelligence-powered-banking/
http://www.bankingtech.com/480262/swedbank-sweet-on-virtual-nina/
https://www.accenture.com/us-en/insight-artificial-intelligence-healthcare
Leave A Comment